Automatic boundary segmentation of vascular Doppler optical coherence tomography images based on cascaded U-net architecture
Abstract—Doppler optical coherence tomography (OCT) imaging of vessels after anastomosis procedures can provide high resolution structure and blood flow imaging of the vessel simultaneously for objective surgical evaluation. Automatic boundary segmentation of the outer vessel wall boundary and its inner lumen contour is a very crucial and fundamental step for the responsive and complicated quantitative analysis required in future clinical applications of Doppler OCT imaging. In this work, we proposed a cascaded U-net (CU-net) architecture to segment the vascular intensity image and its corresponding phase image for the outer vessel wall boundary and the inner blood flowing lumen contour, respectively. CU-net architecture was developed by training two specific U-net frameworks in coordination: the first performs intensity image segmentation while the second performs phase image segmentation. Output of the first framework was sent to the input of the second framework as a mask to select area of interests. Model training time can be reduced effectively by cascading two U-net frameworks. Testing segmentation accuracy for outer vessel wall boundary and inner lumen contour were calculated to be 96.7%±0.2% and 94.8%±0.2%, respectively. The CU-net architecture requires no pre-processing on noises inherent with OCT images, such as random noise and speckle noise. The segmentation was automatic and end-to-end. 250 Doppler OCT images from one in-vivo mouse femoral artery imaging were successfully segmented automatically with an average processing time of 0.68s including both the outer vessel boundary and inner lumen contour. Thrombosis morphology, the inner blood flowing lumen area, and its radius variation were quantitatively analyzed based on the segmentation results, demonstrating the potential for clinical objective evaluation.
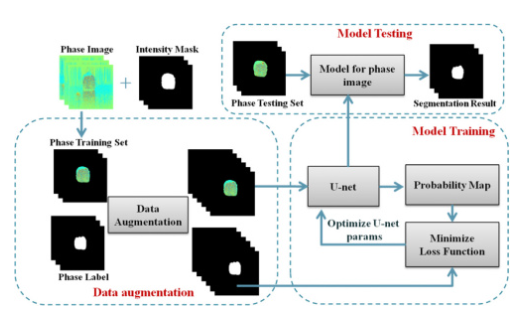