Continuous and complete vascular centerline detection via multi-task attention fusion network (MTAFN).
Abstract—Centerline extraction is significant in coronary reconstruction, lesion detection and surgery navigation. Current pixel-wise classification methods often produce in complete and disconnected vascular map due to the lack of constraint on vessel connectivity and biased centerline localization. In this work, we formulate the centerline extraction as a centerline-based distance transformation(CDT) regression problem, which shows larger central response than conventional boundarybased distance transformation(DT). To enlarge connectivity constraint, vessel direction learning task is appended to provide connectivity contextual information. Moreover, we establish a Multi-task Attention Fusion Network to jointly learn the proposed CDT and vessel direction representation. Notably, the proposed Attention Fusion module concatenates multitask information across different paths and boosts network to converge efficiently. Finally, centerline points correspond to local maximum on learned CDT map at perpendicular vessel direction, which can be easily identified with Non-Maximum Suppression(NMS) algorithm. Experimental results show that our method yields a promising performance on vessel centerline extraction.
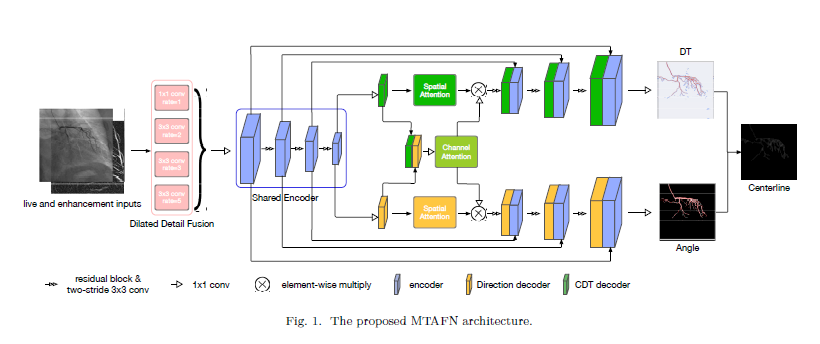