Deep-stacked auto encoder for liver segmentation
Abstract—Deep learning methods have been successfully applied to feature learning in medical applications. In this paper, we proposed a Deep Stacked Auto-Encoder (DSAE) for liver segmentation from CT images. The proposed method composes of three major steps. First, we learned the features with unlabeled data using the auto encoder. Second, these features are fine-tuned to classify the liver among other abdominal organs. Using this technique we got promising classification results on 2D CT data. This classification of the data helps to segment the liver from the abdomen. Finally, segmentation of a liver is refined by post processing method. We focused on the high accuracy of the classification task because of its effect on the accuracy of a better segmentation. We trained the deep stacked auto encoder (DSAE) on 2D CT images and experimentally shows that this method has high classification accuracy and can speed up the clinical task to segment the liver. The mean DICE coefficient is noted to be 90.1% which is better than the state of art methods.
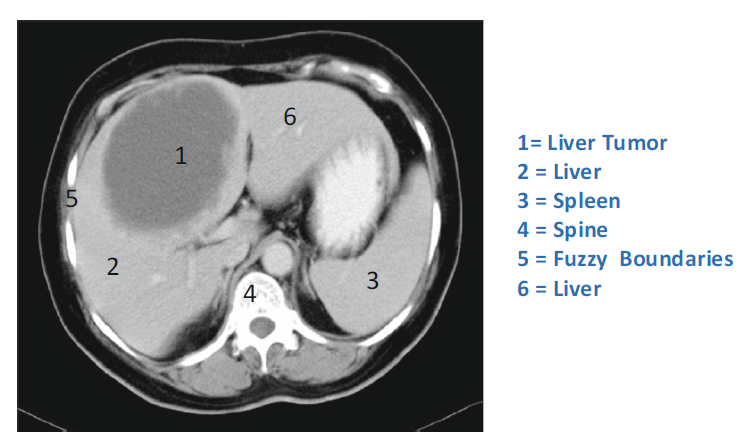