Dial/Hybrid cascade 3DResUNet for liver and tumor segmentation
Abstract—Liver tumor segmentation is an important step in diagnosis of liver cancer. In this work, a cascaded fully convolutional network (FCN) is proposed based on improved 3DResUNet for automatic segmentation of liver and liver tumors. The first FCN is trained to segment the liver as region of interest (ROI) input for the second FCN, which solely segment the tumor from the predicted liver region. Based on 3DResUNet, a Dial-3DResUNet is designed for liver segmentation which combines hybrid dilated convolution to capture global features, a Hybrid-3DResUNet is developed for tumor segmentation, the model consists of Hybrid-3D convolution to effectively extract 3D features while greatly reducing the amount of parameters and decreasing the difficulty of model optimization and the risk of overfitting. Ablation study is conducted on magnetic resonance (MR) data provided by the Chinese PLA General Hospital to demonstrate the effectiveness of the Dial/Hybrid-3DResUNet. In addition, we evaluate our model on 3DIRCADb dataset and achieved a dice global score of 0.958 and 0.742 on liver and liver tumor, respectively.
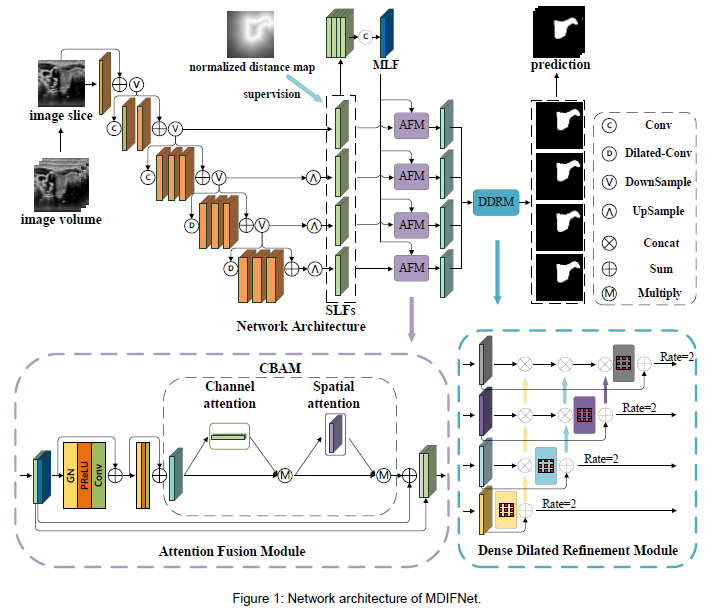