FSNet: Pose estimation of endoscopic surgical tools using feature stacked network
Abstract— Identification of surgical instruments is important to understand surgical scenarios and provide assistant processing in endoscopic image-guided surgery. In this paper, we propose a novel feature stacked network (FSNet) for the recognition of surgical tools in endoscopic images. With a lateral connection and concatenation operation on the different layers of the feature pyramid network, high-level semantic information is fused to low-level features, and the bounding boxes are regressed for the tool instance proposals. Then, low-level semantic information is propagated to a high-level network through the bottom-up feature concatenating path. The keypoints of tools are detected in each proposed boundary box. Two state-ofthe- art end-to-end tool keypoint recognition networks and three backbones are implemented for comparison. The AP and AR of the our FSNet based on ResNeXt101 are 46.1% and 36.5%, respectively, which surpass the results of other methods.
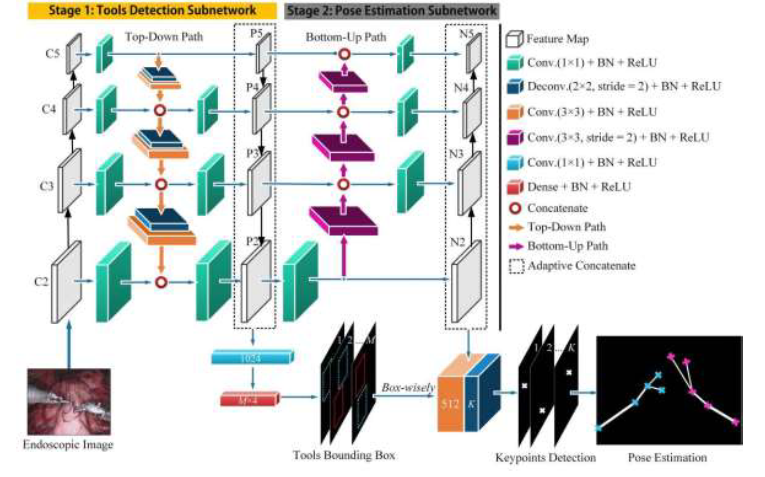