Deep feature descriptor based hierarchical dense matching for x-ray angiographic images
Abstract— Backgroud and Objective: X-ray angiography, a powerful technique for blood vessel visualization, is widely used for interventional diagnosis of coronary artery disease because of its fast imaging speed and per- spective inspection ability. Matching feature points in angiographic images is a considerably challenging task due to repetitive weak-textured regions. Methods: In this paper, we propose an angiographic image matching method based on the hierarchi- cal dense matching framework, where a novel deep feature descriptor is designed to compute multilevel correlation maps. In particular, the deep feature descriptor is computed by a deep learning model specifi- cally designed and trained for angiographic images, thereby making the correlation maps more distinctive for corresponding feature points in different angiographic images. Moreover, point correspondences are further hierarchically extracted from multilevel correlation maps with the highest similarity response(s), which is relatively robust and accurate. To overcome the problem regarding the lack of training samples, the convolutional neural network (designed for deep feature descriptor) is initially trained on samples from natural images and then fine-tuned on manually annotated angiographic images. Finally, a dense matching completion method, based on the distance between deep feature descriptors, is proposed to generate dense matches between images. Results: The proposed method has been evaluated on the number and accuracy of extracted matches and the performance of subtraction images. Experiments on a variety of angiographic images show promising matching accuracy, compared with state-of-the-art methods. Conclusions: The proposed angiographic image matching method is shown to be accurate and effective for feature matching in angiographic images, and further achieves good performance in image subtraction.
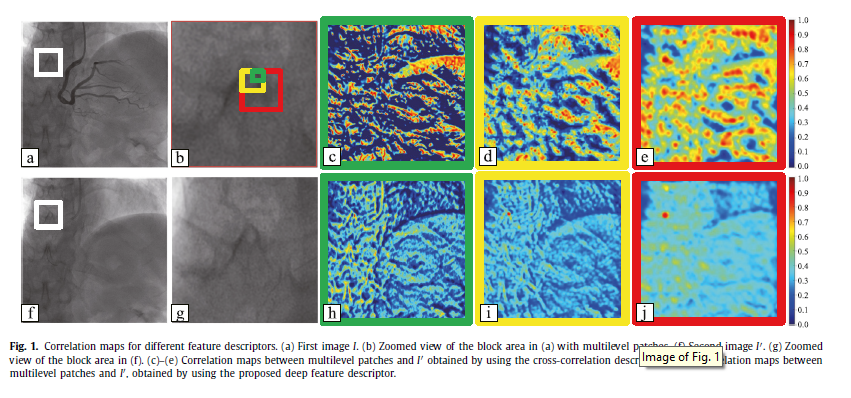