MDIFNet: Multiscale Distant Information Fusion Network for Thyroid Segmentation in 3D Ultrasound Image
Abstract—The thyroid segmentation in 3D ultrasound image can provide necessary volumetric information in diagnosis and treatment. Clinically, 3D thyroid US images are usually stacks of 2D frames acquired by freehand scanning, which results in good performance of 2D segmentation methods. However, thyroid nodules cause difficulty to these algorithms because of strong anisotropy, whereas current approaches are mostly for healthy thyroid images. This paper proposes a multiscale distant information fusion network to segment clinical thyroid images with nodules. Our fully convolutional network consists of the following: (1) deep supervision of normalized boundary distance map, (2) multi-scale fusion module combined with attention mechanism, and (3) dense dilated refinement module to refine segmentation probability in a multi-scale way. We conducted ablation study and cross-validation on clinical dataset provided by The Third Affiliated Hospital of Sun Yat-Sen University and obtained the best dice result of 0.9070. Notably, our method also outperformed other methods on ultrasonography dataset and obtained a dice score of 0.93.
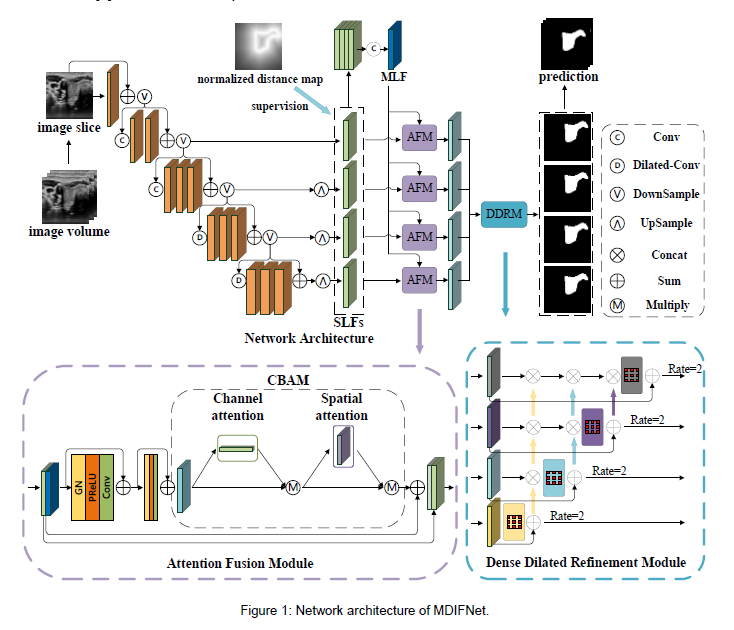