Few-shot learning for deformable medical image registration with perception-correspondence decoupling and reverse teaching
Abstract—Deformable medical image registration estimates corresponding deformation to align the regions of interest (ROIs) of two images to a same spatial coordinate system. However, recent unsupervised registration models only have correspondence ability without perception, making misalignment on blurred anatomies and distortion on task-unconcerned backgrounds. Label-constrained (LC) registration models embed the perception ability via labels, but the lack of texture constraints in labels and the expensive labeling costs causes distortion internal ROIs and overfitted perception. We propose the first fewshot deformable medical image registration framework, Perception-Correspondence Registration (PC-Reg), which embeds perception ability to registration models only with few labels, thus greatly improving registration accuracy and reducing distortion. 1) We propose the Perception Correspondence Decoupling which decouples the perception and correspondence actions of registration to two CNNs. Therefore, independent optimizations and feature representations are available avoiding interference of the correspondence due to the lack of texture constraints. 2) For few-shot learning, we propose Reverse Teaching which aligns labeled and unlabeled images to each other to provide supervision information to the structure and style knowledge in unlabeled images, thus generating additional training data. Therefore, these data will reversely teach our perception CNN more style and structure knowledge, improving its generalization ability. Our experiments on three datasets with only five labels demonstrate that our PC-Reg has competitive registration accuracy and effective distortion-reducing ability. Compared with LCVoxelMorph( λ = 1), we achieve the 12.5%, 6.3% and 1.0% Reg-DSC improvements on three datasets, revealing our framework with great potential in clinical application.
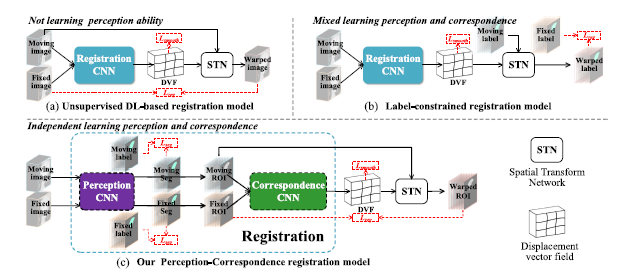