Towards Personalized Deformable and Mix-supervised Model for Robust MR-US Registration
Abstract—Registration and fusion of magnetic resonance (MR) and ultrasound (US) images remain challenging tasks due to the great intensity variation between two modalities and the lack of ground truth spatial correspondence. In this paper, we proposed a mix-supervised registration network, driven by main vessel segmentation labels and modality independent neighborhood descriptor (MIND) similarity metric. The accurate alignment of the main vessel labels can prompt the registration network focus on the region of interest and MIND similarity metric constraints global deformation. Vessel label and similarity measures can promote each other and produce satisfactory registration results. This framework connects a Rigid-Net and a Bspline-Net to generate the transformation parameters of rigid and deformable registration in one shot. The well-trained network is able to register dynamic 3D US images to the preoperative MR image in real time, correcting the motion caused by a patient’s breathing. Experimental results show that the proposed method is effective for MR-US registration.
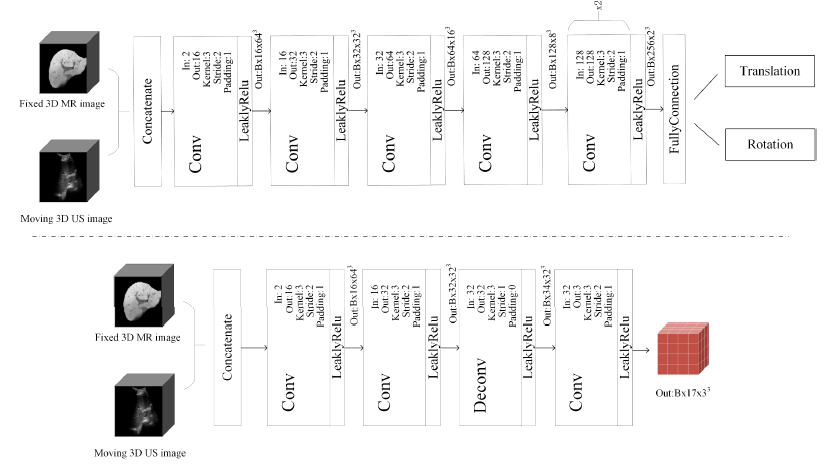