Multi-level feature aggregation network for instrument identification of endoscopic image
Abstract—Identification of surgical instruments is crucial in understanding surgical scenarios and providing an assistive process in endoscopic image-guided surgery. This study proposes a novel multilevel feature-aggregated deep convolutional neural network (MLFA-Net) for identifying surgical instruments in endoscopic images. First, a global feature augmentation layer is created on the top layer of the backbone to improve the localization ability of object identification by boosting the high-level semantic information to the feature flow network. Second, a modified interaction path of cross-channel features is proposed to increase the nonlinear combination of features in the same level and improve the efficiency of information propagation. Third, a multiview fusion branch of features is built to aggregate the location-sensitive information of the same level in different views, increase the information diversity of features, and enhance the localization ability of objects. By utilizing the latent information, the proposed network of multilevel feature aggregation can accomplish multitask instrument identification with a single network. Three tasks are handled by
the proposed network, including object detection, which classifies the type of instrument and locates its border; mask segmentation, which detects the instrument shape; and pose estimation, which detects the keypoint of instrument parts. The experiments are performed on laparoscopic images from MICCAI 2017 Endoscopic Vision Challenge, and the mean average precision (AP) and average recall (AR) are utilized to quantify the segmentation and pose estimation results. For the bounding box regression, the AP and AR are 79.1% and 63.2%, respectively, while the AP and AR of mask segmentation are 78.1% and 62.1%, and the AP and AR of the pose estimation achieve 67.1% and 55.7%, respectively. The experiments demonstrate that our method efficiently improves the recognition accuracy of the instrument in endoscopic images, and outperforms the other state-of-the-art methods.
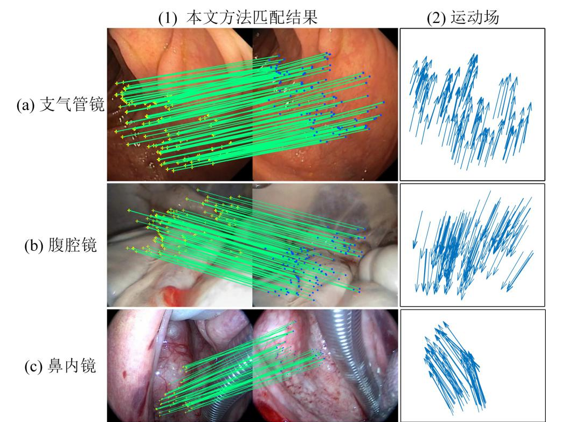